Transforming Supply Chains with ML: A Modern Enterprise Revolution
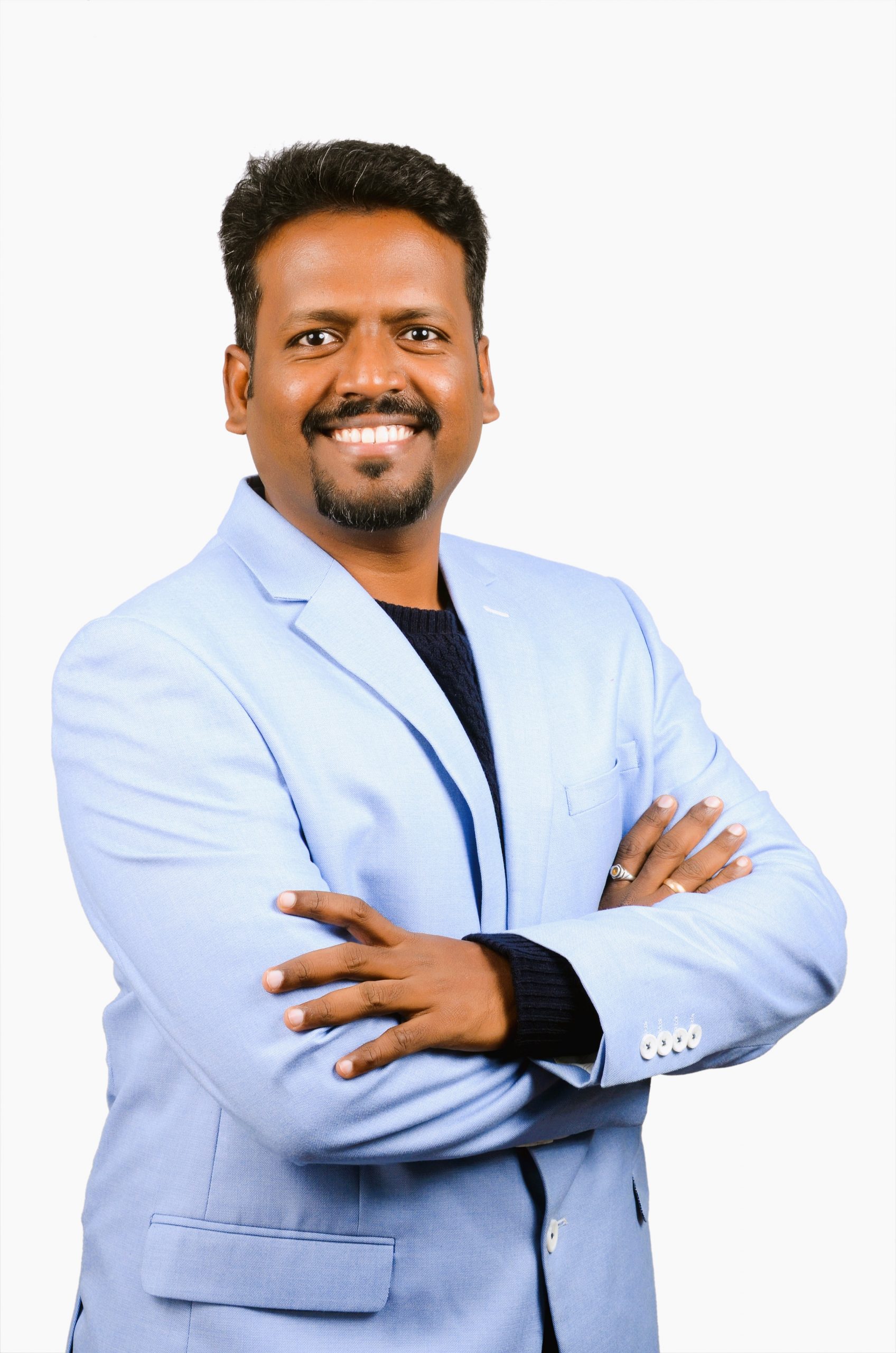
Client Partner, Pearson NA
Swift AI Integration and Deployment with Quixl, AI accelerator. Request a Demo
Client Partner, Pearson NA
In today’s hyper-competitive landscape, efficient supply chain operations are no longer a luxury, but a strategic imperative for modern enterprises. They directly impact factors like cost, responsiveness, and ultimately, customer satisfaction. However, traditional approaches often struggle with siloed data, limited visibility, and reactive decision-making. Enter the game-changer: advanced Machine Learning (ML) models. The machine learning market is expected to grow from around 140 billion U.S. dollars to nearly two trillion U.S. dollars by 2030. By harnessing the power of data and intelligent algorithms, ML can unlock unprecedented levels of optimization and agility in your supply chain.
Traditional methods frequently face obstacles like:
These challenges directly impact business performance, leading to lost revenue, increased costs, and dissatisfied customers.
Advanced ML models can address these challenges head-on by:
Amazon SageMaker focuses on machine learning-based business practices. It uses terabytes of data from the Amazon databases and helps them to eliminate all the waste of packaging that is done by predicting the user buying forecast and then identifying which of the smaller products can be shipped in a small paper bag and require less packaging in order to reduce all the waste packaging.
More Retail Ltd. (MRL) is one of India’s top four grocery retailers, with a revenue in the order of several billion dollars. MRL used Amazon Forecast to increase their forecasting accuracy from 24% to 76%, leading to a reduction in wastage by up to 30% in the fresh produce category, improving in-stock rates from 80% to 90%, and increasing gross profit by 25%.
While the benefits of ML in supply chain optimization are evident, the implementation process can seem daunting. Here’s a step-by-step guide to help you navigate it effectively:
1. Assess Your Needs and Data:
2. Choose the Right ML Model and Tools:
3. Start Small and Scale Up:
4. Build Your Team and Expertise:
5. Remember the Human Factor:
The future of ML in supply chain management is bright, with emerging trends like:
By embracing advanced ML models, enterprises can unlock the full potential of their supply chains, achieving greater efficiency, cost reduction, and agility. The journey starts with acknowledging the challenges, understanding the power of ML, and taking the first step towards data-driven optimization. The future of supply chain management is intelligent, automated, and data-driven, and those who embrace this change will be the ones leading the pack. Feel free to connect with our team to learn more about custom ML models and how you can incorporate them in your business.
Peer review is vital to ensure research integrity. Learn how it ensures credible findings and the emerging role of AI in enhancing the academic publishing process...more
In today’s fast-evolving business landscape, AI business intelligence stands at the forefront of revolutionizing data analysis and strategic decision-making processes. By harnessing the power of advanced algorithms and machine learning, businesses are able to unlock valuable insights from vast amounts of data more efficiently than ever before. This shift not only enhances operational efficiency but […]..more
Explores how AI-powered proofing tools significantly enhance author productivity by automating repetitive tasks. From real-time grammar checks to seamless collaboration, discover how AI-driven solutions are transforming content creation and driving faster, higher-quality publications. ..more
The business landscape is in a state of constant flux, demanding innovation and agility. Artificial intelligence (AI) has emerged as a powerful tool for organizations seeking to transform their operations, optimize processes, and gain a competitive edge. A recent McKinsey report cites that up to 20% of a company’s EBIT (earnings before interest and taxes) […]..more
Uncover how generative AI is reshaping business landscapes by enhancing decision-making capabilities. This blog explores the practical applications, benefits, and strategic integration of GenAI and API models in modern business operations. ..more
Explore the transformative power of AI automation in the finance sector, from fraud detection to high-frequency trading, and learn how it minimizes errors while maximizing efficiency. ..more
© 2023 | Integra Software Services Pvt. Ltd.