A Step-by-Step Guide to Implementing AI-Based Automation in Your Workflow
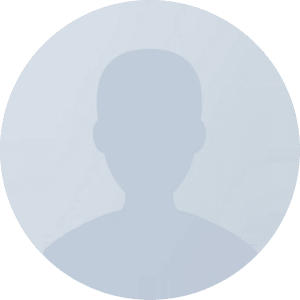
Lead Programmer
Swift AI Integration and Deployment with Quixl, AI accelerator. Request a Demo
Lead Programmer
The technological spectrum is rapidly shifting towards a paradigm where AI-based automation is at the forefront. Not only does it promise operational efficiency, but it also paves the way for businesses to remain agile in a competitive landscape. Integrating AI into workflows today is not just a trend; it is an imperative step for those looking to redefine and upgrade their operational strategies. And that is why intelligent process automation (IPA) market is forecast to expand globally with a CAGR of 14.6% between 2023 and 2032.
AI-based automation refers to systems or tools that utilize artificial intelligence (AI) to perform tasks without human intervention. Unlike traditional automation that follows predefined rules, AI-driven automation can learn, adapt, and improve over time. Machine learning held 40% of the total AI market share in 2022, for its adoption and forms the foundation of IPA. The essence of this modern automation lies in its efficiency, precision, scalability, and adaptability. It has the prowess to analyze vast data sets swiftly, making real-time decisions, scaling as per demands, and evolving through learning. This has transformed its application as just a cost-saving lever to a tool to create new revenue streams. That is why 69% of respondents in PR Newswire’s study affirmed that their organizations had at least 1 AI project in production.
Before delving into AI automation, certain prerequisites need to be in place. A robust infrastructure comprising computing resources, pertinent software, and seamless data pipelines is essential. Moreover, the human touch can’t be ignored. A deep understanding of data science, familiarity with relevant algorithms, and domain-specific knowledge are vital. Above all, the quality and relevance of data can’t be overstated. Ensuring that the data is representative, free from biases, and appropriately preprocessed sets the stage for successful AI integration.
Begin by mapping out tasks that are repetitive and time-consuming. With a clear view, assess which of these tasks can be automated and the potential return on investment (ROI) of doing so.
Numerous platforms cater to different automation needs. While most AI tools are powerhouses for deep learning, niche tools provide a more straightforward approach for those without deep technical expertise. Factors such as scalability, community support, ease-of-use, and ongoing development should guide your choice.
The foundation of any AI system is data. It’s imperative to gather diverse and representative data sets. Once collected, the data should be cleaned, normalized, and split (typically into training, validation, and test sets) to pave the way for model development.
Based on the task at hand, select the appropriate algorithms. Train the model using the prepared data sets, adjusting hyperparameters as necessary to enhance its performance.
Once trained, test the model against a set of unseen data. This phase helps in understanding its real-world efficacy. Based on the outcomes, refinements can be made to further improve accuracy and reliability.
Transitioning a trained model into a tool that integrates seamlessly into your workflow is crucial. Ensure that this integration is smooth, with minimal disruptions to existing processes.
AI models, once deployed, are not set in stone. They should be continuously monitored for performance. Feedback loops are vital to ensure the model stays updated and relevant to the changing data or environment.
Like any technological implementation, AI-based automation isn’t without its challenges. Here’s what a study by PR Newswire highlighted:
One significant challenge is the potential for skewed or biased data, which can lead to unfair or incorrect outcomes. Ensuring transparency and explainability in AI models is vital to build trust. Furthermore, as data is the backbone of AI, maintaining its privacy and security becomes paramount.
AI-based automation holds a transformative potential that can redefine the operational capacities of businesses across the spectrum. The future is automated, and AI is leading the way. The technology is expected to add $15.7 trillion to the global economy by 2030. Embracing AI isn’t about making a massive leap but taking systematic, informed steps. Start small, understand the nuances, iterate based on feedback, and refine your approach continuously.
The pandemic underscored the strategic importance of learning and development (L&D) within corporate structures, with 62% of business leaders recognizing it as either highly important or critical to business success. Yet, despite this recognition, a persistent challenge remains. Before and during the pandemic, 55% of organizations reported an inability to effectively measure the impact of […]..more
Discover how iLancer streamlines freelance operations with centralized project management, communication tools, and automated payment systems...more
The European Accessibility Act – Promoting Digital Inclusivity Digital accessibility is increasingly acknowledged as a fundamental human right, vital for social inclusion and equal opportunity. The European Accessibility Act (EAA) spearheads initiatives to ensure that all digital products and services are accessible, particularly for individuals with disabilities. As a cornerstone of legislative efforts towards inclusivity, […]..more
Explore the profound impact of AI on the media and entertainment sector, from revolutionizing content production to redefining customer interactions...more
As artificial intelligence (AI) evolves, its resonance spreads across various sectors, notably in the publishing industry. This article explores the dynamic interplay between human linguistic expertise and AI-driven, language models, focusing on how their integration reshapes publishing. We examine both the technical aspects and the practical applications of AI in publishing, alongside the intrinsic human […]..more
The landscape of modern business is constantly evolving. Artificial intelligence (AI) automation is rapidly transforming how companies operate, automating repetitive tasks, streamlining processes, and unlocking new levels of efficiency. While the financial benefits of AI automation are undeniable, a truly comprehensive understanding of its return on investment (ROI) requires looking beyond the bottom line. According […]..more
© 2023 | Integra Software Services Pvt. Ltd.